QMUL-SurvFace: Surveillance Face Recognition Challenge
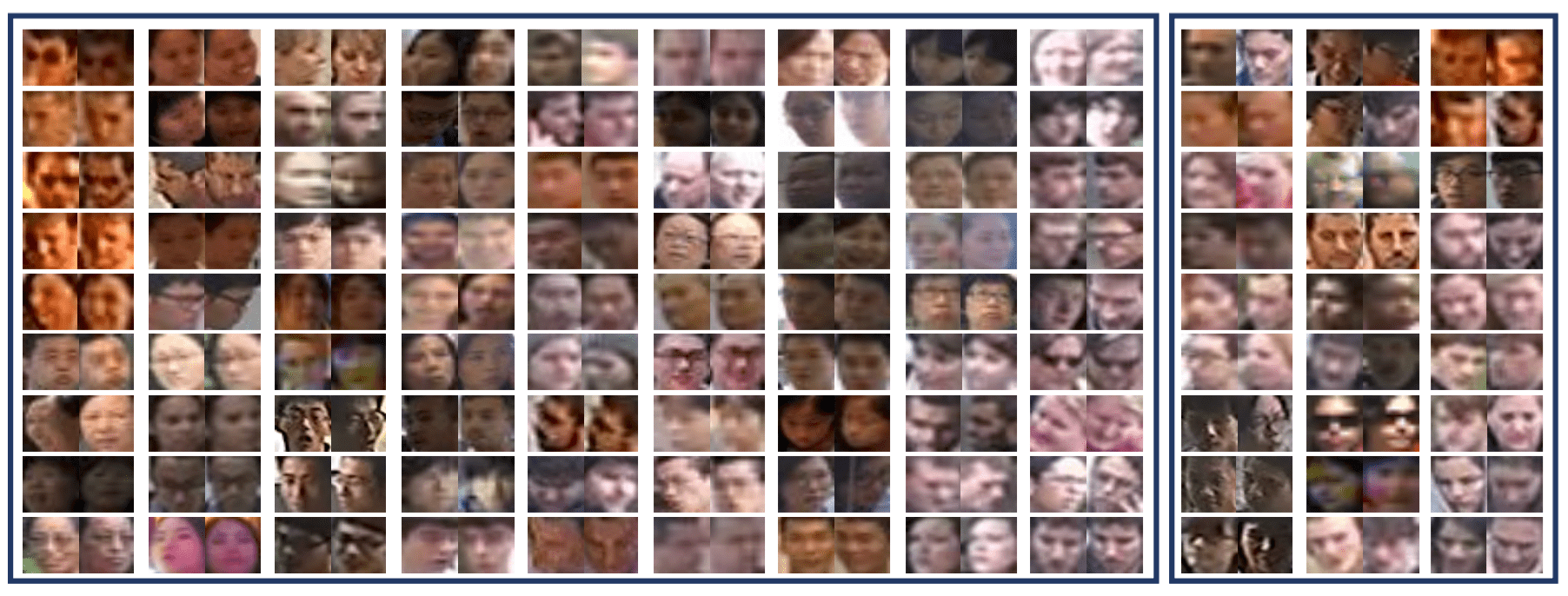
Description
To facilitate more studies for developing face recognition methods that are effective and robust against low-resolution surveillance facial images, a new Surveillance Face Recognition challenge, QMUL-SurvFace, is introduced. This new challenge is the largest and more importantly the only true surveillance face recognition benchmark to our best knowledge, where low-resolution face images are native and not synthesised by artificial down-sampling of native high-resolution images. This challenge contains 463,507 face images of 15,573 distinct identities captured in real-world uncooperative surveillance scenes across wide space and time. Face recognition is generally more difficult in an open-set setting which is typical for surveillance person search scenarios, owing to an arbitrarily large number of non-target people (distractors) appearing over open space and unconstrained time.
News
- May 28, 2019: We are organizing the QMUL-SurvFace: Surveillance Face Recognition Challenge in conjunction with the ICCV'19 Workshop and Challenge on Real-World Recognition from Low-Quality Images and Videos (RLQ) .
- August 29, 2018: Updated the evaluation results of the state-of-the-art face recognition methods.
- June 01, 2018: QMUL-SurvFace dataset, the evaluation protocol, and test codes are released.
Download
QMUL-SurvFace Dataset and Evaluation Codes (389MB): [Google Drive] [Baidu Cloud]
Citation
Surveillance Face Recognition Challenge. Zhiyi Cheng, Xiatian Zhu and Shaogang Gong. Technical Report, 2018. Paper Bibtex
Related Datasets
We list below existing surveillance face recognition datasets. More extensive comparisons of face recognition datasets can be found in the paper.
- UCCS Challenge: UCCS is a high-resolution surveillance face detection and recognition challenge. It contains 1,732 identities captured by a Canon 7D camera fitted with Sigma 800mm F5.6 EX APO DG HSM lens.
- SCface Dataset: SCface is one of the earliest surveillance face recognition datasets. It consists of 4,160 static images (in visible and infrared spectrum) of 130 identities.
Licence
Notice that the QMUL-SurvFace challenge is made available for research purposes. All the images were collected from the existing person re-identification datasets, and the copyright belongs to the original owners.
Contact
Please feel free to send any questions, comments, and evaluation results with a brief method description to Zhiyi Cheng at z.cheng@qmul.ac.uk.